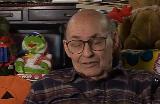
May the 7th, 2024: xLSTM - Extended Long Short-Term Memory
"May the 4th be with ...", aber 7 ist auch eine feine Zahl.
http://www.dma.ufg.ac.at/assets/25937/intern/Sepp_Hochreiter_xLSTM.mp3
Das Unternehmen NXAI aus Österreich will das schnellste und beste LLM der Welt bauen. (Austria, Linz)
xLSTM: A European Revolution in Language Processing Technology
Welcome to the forefront of artificial intelligence and language processing innovation — introducing xLSTM. Developed by the visionary AI mastermind, Sepp Hochreiter, in collaboration with NXAI and the Johannes Kepler University Linz, xLSTM sets a new standard in large language models (LLMs) by offering significantly enhanced efficiency and performance in text processing.
Innovating at the Heart of Europe
Sepp Hochreiter, a pioneer in artificial intelligence, has once again broken new ground with the development of xLSTM. This European LLM not only continues his tradition of innovation, dating back to his invention of Long Short Term Memory (LSTM) technology in 1991, but it also marks a significant leap forward. While LSTM technology laid the groundwork for modern AI, remaining the dominant method in language processing and text analysis until 2017, xLSTM propels this legacy into the future with cutting-edge advancements.
The xLSTM Difference: Efficiency Through Innovation
Ever since the early 1990s, the Long-Short Term idea of constant error carousel and gating has contributed to numerous success stories. LSTMs have emerged as the most performant recurrent neural network architecture with demonstrated efficacy in numerous sequence-related tasks. When the advent of the Transformer technology with parallelizable self-attention at its core marked the dawn of a new era, the LSTM idea seemed outpaced. Transformers have become the driving force of today’s LLMs, piggy-backing the modern trend of ever-improving compute and ever-increasing data. At NXAI, we put the LSTM idea back into the game, but more powerful than ever. We build on the unique expertise lead by LSTM inventor Sepp Hochreiter, and introduce xLSTM, the new asset on the LLM market.
NXAI: Accelerating the LLM Revolution
Early results from Sepp Hochreiter's research team illustrate that xLSTM operates more efficiently, using less computing power, and outperforms current LLMs in speed and accuracy. Notably, xLSTM demonstrates a superior understanding of text semantics, enabling it to comprehend and generate more complex texts. This makes xLSTM a key component in the development of large AI-driven surrogates, with NXAI providing the essential platform for this innovation.
A Vision from Sepp Hochreiter
"xLSTM represents more than just a technological breakthrough; it is a step towards a future where the efficiency, accuracy, and understanding of language processing can meet and exceed human capabilities."
For researchers and investors alike, xLSTM offers a glimpse into the future of artificial intelligence, where innovation and efficiency pave the way for new possibilities. Join us in this exciting journey as we explore the potential of xLSTM and its impact on the world of AI.
_____________________________
xLSTM: Extended Long Short-Term Memory
xLSTM: Extended Long Short-Term Memory
Designed to shape the future, xLSTM turns back the years. xLSTM revives the Long Short-Term Memory (LSTM) idea, i.e., concept of constant error carousel and gating. Introduced by Sepp Hochreiter and Jürgen Schmidhuber LSTM is a revolutionary 1990s Deep Learning architecture that managed to overcome the vanishing gradient problem for sequential tasks such as time series or language modeling. Since then, LSTM has stood the test of time and contributed to numerous Deep Learning success stories, in particular they constituted the first Large Language Models (LLMs). However, the advent of the Transformer technology with parallelizable self-attention at its core marked the dawn of a new era, outpacing LSTM at scale.
NXAI is funded to raise a simple question: How far do we get in language modeling when scaling LSTMs to billions of parameters, leveraging the latest techniques from modern LLMs?
To answer this question, our team lead by Sepp Hochreiter had to overcome well-known limitations of LSTM, namely the inability to revise storage decisions, limited storage capacities, and lack of parallelizability. In this vein, our team introduces exponential gating with appropriate normalization and stabilization techniques. Secondly, our team equips xLSTM with new memory structures, and consequently obtain: (i) sLSTM with a scalar memory, a scalar update, and new memory mixing, (ii) mLSTM that is fully parallelizable with a matrix memory and a covariance update rule. Integrating these LSTM extensions into residual block backbones yields xLSTM blocks that are then residually stacked into xLSTM architectures.
Those modifications are enough to boost xLSTM capabilities to perform favorably when compared to state-of-the-art Transformers and State Space Models, both in performance and scaling. The results reported in our paper indicate that xLSTM models will be a serious competitor to current Large Language Models.
xLSTM is our innovative new building block, i.e., the heart of a new wave of European LLMs, which we are developing in house here at NXAI.
xLSTM: Extended Long Short-Term Memory
Maximilian Beck, Korbinian Pöppel, Markus Spanring, Andreas Auer, Oleksandra Prudnikova, Michael Kopp, Günter Klambauer, Johannes Brandstetter, Sepp Hochreiter